Give it in plain text: Making your content AI-Ready
Written by Knut Melvær
I find myself peer-programming with LLMs more often, especially when I want to quickly bring an idea to life or add a minor feature to a code base. AI-powered coding really shines when it comes to exploring ideas and getting something that runs quickly off the ground.
The other day, I watched an AI bootstrap a new Astro + Sanity blog in about a minute (yes, I timed it - professional curiosity and all). It was impressive, but like many quick solutions, it wasn't quite what we'd recommend in our developer education materials. It missed our official Astro integration, skipped proper TypeGen setup, and the content model was, well, let's say it needed some of our hard-earned structured content wisdom.
This got me thinking about a bigger question: how do we ensure AI tools can access and understand our educational content the same way developers do? The answer turned out to be deceptively simple, but getting there? That's the story I want to share.
When "the most likely" isn't what you want
Here's the thing about LLMs: they're like that friend who's read every programming blog post ever written but hasn't actually worked on your specific project. They'll give you the most likely patterns based on what they've seen across GitHub repositories and blog posts. And while that's often good enough, it's not always what we'd call "the Sanity way."
To be completely honest, we haven't been able to share everything we've learned from helping customers and figuring out these patterns ourselves over the years. This can leave developers in a bit of a pickle if they rely too heavily on LLM-generated code without guidance.
This isn't just our problem - it's a challenge for anyone building developer tools in our AI-enhanced world:
- The models learned from what's out there, including that Stack Overflow answer from 2019 that everyone keeps copying
- The generated code works, but might not follow the best practices we've discovered since then
- And unless you explicitly tell them, these models won't know about that cool new feature you just shipped last week
This is why AI-powered code editors like Cursor have features to quickly add a documentation site to its context.
Enter the "Agent Experience"
More broadly, this is also why user experiences in front of LLMs, like ChatGPT, increasingly go out on the web to bring more context into their prompts and output better and more accurate information.
And this is where the "Agent Experience" concept comes in handy. Coined by Mathias Biilmann, Founder/CEO of Netlify, in the blog post “Introducing AX: Why Agent Experience Matters”:
Is it simple for an Agent to get access to operating a platform on behalf of a user? Are there clean, well described APIs that agents can operate? Are there machine-ready documentation and context for LLMs and agents to properly use the available platform and SDKs? Addressing the distinct needs of agents through better AX, will improve their usefulness for the benefit of the human user.
I had been wrestling with this exact challenge a week before Matt's post. I wanted a straightforward way to feed all our learning platform content into Claude (and between you and me, I'm not entirely sure how good ChatGPT's web search is at getting all the content either).
A recent Vercel analysis of AI crawlers showed they're still finding their feet - they don't render JavaScript, are picky about content types, and tend to stumble around your site like a tourist without a map. We needed something better.
So, how do I go about this? The answer might not surprise you.
llms.txt: Like devs, agents love plain text too
As I'm writing this, there is a conversation about how best to accommodate agents visiting your site, provided that you want to make your content accessible. There seems to be a growing consensus around giving them content such as plain text and markdown.
In our opinion, Markdown is not a great format for storing content (you can read my 6000 words about why here, or just read this short summary), but it turns out to be great as a format to interface with LLMs (that has been trained on a lot of Markdown syntax).
The jury is still out on the conventions of making the plain text accessible, but one pattern seems to catch on. /llms.txt
is proposed by the folks at Answer.ai, but there are also discussions on using the /.well-known/llms.txt
IANA proposal. The documentation platform Mintlify has launched /llms.txt
as a feature, as has Anthropic, Svelte, and Vercel's AI SDK for their documentation.
They generally seem to use this pattern for exposing content as plain text:
/llms.txt
is an abbreviated index of all the content with links/llms-small.txt
is the abbreviated content for smaller context windows-
/llms-full.txt
is the complete content (sometimes optimized to fit within the context window limits)
Beyond Plain Text: The Structured Content Advantage
While converting content to plain text formats like /llms.txt
provides a solid starting point for AI consumption, this approach has inherent limitations that echo the challenges developers face when working with unstructured content.
Plain text dumps have an undeniable simplicity - they're straightforward to implement and universal in compatibility. However, context is precious real estate. Using it inefficiently means slower queries and potentially less relevant responses. When developers paste thousands of tokens into a model's context window, that information needs to deliver significant value to justify its inclusion.
The plain text approach also fails to capture the rich relationships and metadata that make content truly valuable. LLMs can produce functional code that works, but may miss nuanced best practices like using named exports instead of default exports, implementing proper TypeScript definitions, or applying organization-specific patterns that have evolved through hard-won experience.
This is where Sanity's structured content approach offers significant advantages:
- Relationship-aware content: Unlike plain text, structured content understands that a blog post can have multiple authors, that images have alt text, and that references connect related pieces of content together.
- Queryable knowledge: With a structured approach, models could potentially query exactly what they need rather than processing the entire documentation corpus for every request.
- Contextual best practices: Structured content can encode not just what something is, but how it should be used according to established patterns and practices.
- Evolving knowledge representation: As your product and best practices evolve, structured content provides a framework for updating knowledge representations without rebuilding from scratch.
The future likely isn't about choosing between plain text or structured content for AI consumption, but rather about creating intelligent interfaces that leverage structured content's richness while maintaining the accessibility of plain text formats. Just as we've developed sophisticated serializers that transform Portable Text into React components, we need similar approaches that can present our structured content to AI in ways that preserve its semantic meaning.
We'll likely see conventions for agent-specific context emerging soon - not just turning content into flat text, but creating rich, contextual metadata layers that help AI systems navigate and utilize content more effectively.
The /llms.txt
approach is a practical starting point, but just as web development has evolved beyond static HTML to component-based architectures, AI content consumption will likely follow a similar trajectory toward more structured, semantic representations.
Now, enough with the think piece, let's look at how we brought this pattern to Sanity Learn.
Building a plain text route for Sanity Learn
Our learning platform is built with React Router 7 formerly known as Remix, and Sanity (naturally), with lesson content stored in Portable Text fields. We have custom blocks and marks for the different learning affordances (tasks, code blocks, callouts, etc). Portable Text stores this block content as JSON, which makes it queryable and provides neat integration in front end frameworks because it lets you directly serialize content as props to your components.
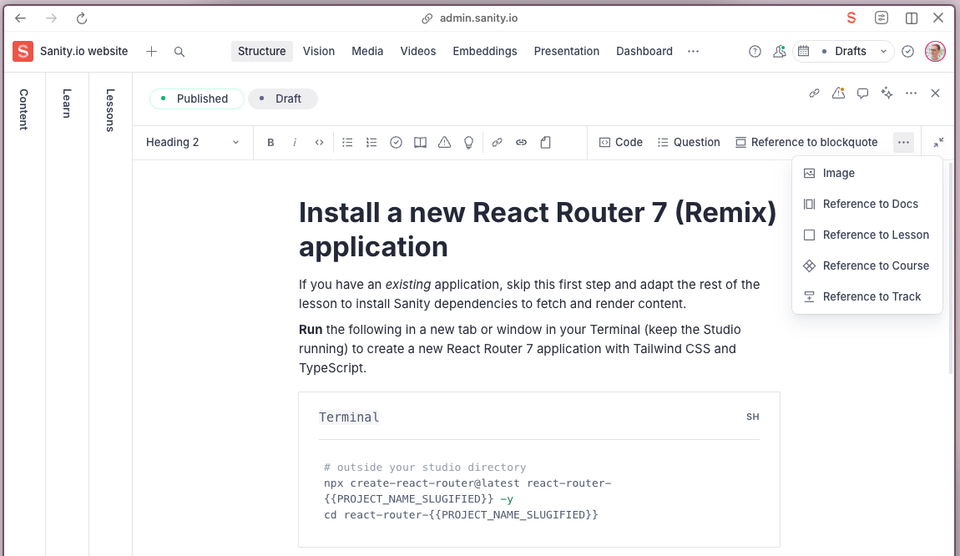
Here is an example of how Portable Text serialization to React looks like:
Internal server error